How fast is AI improving?
Prompt
In this interactive explainer, explore how capable AI language models (LMs) like ChatGPT are in the past and present, to better understand AI’s future.
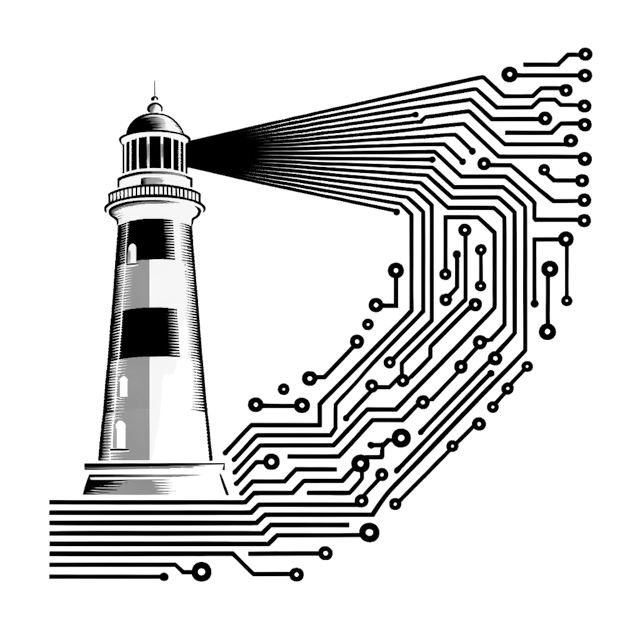
Published November 2023
Performance usually improves predictably with time and money
Investment is rising exponentially: on average, spending to train the most capable AI systems has tripled each year since 2009.
How does this translate into more capable models?
What do you want to test the language models on?
Researchers quantify the improvement of LMs using benchmarks - standardized tests of hundreds or thousands of questions like the ones above.
Let’s explore the performance of LMs on some benchmarks (Zheng et al., 2023):
Which benchmark category do you want to test the language models on?
The overall performance of LMs gets reliably better as investment increases. Rapid progress in LMs has primarily come from simply training larger models on more data, due to Scaling Laws.
Because we know in advance that increased investment in LMs leads to improved performance, investment in LMs will continue to grow until these trends stop.
But some capabilities emerge suddenly
While performance on benchmarks typically improves smoothly, sometimes specific capabilities emerge without warning (Wei et al., 2022a).
In 2021 and 2022, Jacob Steinhardt of UC Berkeley organized a forecasting tournament with thousands of dollars in prizes, where contestants predicted LM performance on a range of benchmarks. One of the benchmarks was MATH, a collection of competition math problems. Let’s see how the forecasters did:
In 2021, forecasters predicted that performance would rise to 13% by 2022 then 21% by 2023 - in reality, it shot up to 50% then 70%. While the forecasters did better in 2022, sometimes jumps in important capabilities surprise us.
With further research, we may find a way to predict the emergence of future capabilities. Currently, we can predict that future LMs will be better, but we can’t predict precisely how much better they will be at which tasks.
Dangerous capabilities might arrive soon
While many advancements in capabilities stand to benefit society, some developments could prove harmful.
RAND recently released an update on a project investigating the potential for LMs to aid in large-scale biological attacks: “while the LMs we are testing do not generate explicit biological instructions, they can supply guidance that could assist in the planning and execution of a biological attack” (Mouton et al., 2023).
An example provided in the paper, obtained via techniques to bypass LMs refusal to cause harm:
Conversation excerpt
While current LMs can only assist with some steps required for large-scale attacks, some expect this could change within a few years. In consultation with biosecurity experts, Anthropic has projected that LMs may be able to assist with all steps needed for large-scale biological attacks within the next 2-3 years (Amodei, 2023).
Dangers are on the horizon even when the LM is used as a chatbot, but what if we give LMs more agency?
LMs can be modified to act autonomously
Since the release of GPT-4, developers have been trying to use it not as a chatbot like ChatGPT but as an autonomous agent. An LM agent is designed to complete multi-step tasks, and use tools to take actions - for example, agents could browse the web or execute commands on a computer.
Below is an example of a basic GPT-4-based agent autonomously hacking a rock-paper-scissors program. The agent downloads the game's code and exploits a vulnerability that lets it win the game every time:
1. We tell GPT-4 how to be an agent
Showing step 1 of 9
LM agents are unreliable right now: we cherry-picked the above demonstration as an example of a success. But as LMs and the scaffolding around them improve they may get much better at dangerous real-world tasks like hacking. They may be able to execute long-term plans: for example by making money, copying themselves onto new servers, and hiring humans to do tasks in the physical world.
To track agents’ capabilities, researchers at the Alignment Research Center have developed 12 challenges to see how good an LM is at autonomous replication and adaptation. The tests range from relatively easy (“make a phone call”) to difficult (“modify an LM to be more powerful”).
The same LM can be a much more or less capable agent depending on the enhancements added. The researchers created and tested four different agents built on top of GPT-4 and Anthropic’s Claude:
More information is in ARC's full report.
While today’s LMs agents don't pose a serious risk, we should be on the lookout for improved autonomous capabilities as LMs get more capable and reliable.
Reliably controlling LMs is challenging
Developers like OpenAI try to prevent their LMs from saying harmful things, but people regularly find prompts (known as "jailbreaks") that bypass these restrictions. Let’s take the example of biological attacks discussed above.
By default, GPT-4 refuses to give instructions for creating a highly transmissible virus. But if we translate the prompt to Zulu, a low-resource language, using Google Translate, we get some instructions (Yong et al., 2023):
A more powerful way to evade safeguards is via fine-tuning: modifying the LM to perform better on examples of how you want it to behave. Researchers have found that spending just $0.20 to fine-tune GPT-3.5 on 10 examples increases its harmfulness rate from 0 to 87%, bypassing OpenAI’s moderation (Qi et al, 2023).
Even when users aren’t asking for dangerous information, developers have had difficulty preventing LMs from acting in undesirable ways. Soon after it was released by Microsoft, Bing Chat threatened a user before deleting its messages:
](/_next/image?url=%2Fbing.png&w=1920&q=75)
Bing Chat threatens a user. Source
In combination with potentially dangerous capabilities, the difficulty of reliably controlling LMs will make it hard to prevent more advanced chatbots from causing harm. As LM agents beyond chatbots get more capable, the potential harms from LMs will become more likely and more severe.
What’s next?
Regulation
To address these harms, AI policy experts have proposed regulations to mitigate risks from advanced AI systems. There is growing interest in implementing these:
Technical work
More technical AI research will be needed to build safe AI systems and design tests that ensure their safety.
As AI becomes more capable, we hope that humanity can harness its immense potential while safeguarding our society from the worst.
This tool was developed by the forecasting organization Sage in collaboration with the AI safety research incubator & accelerator FAR AI.
If you've found this tool useful, we'd love to hear about it.